Education is in the grip of a paradox.
As educators, we are responsible for addressing the individual needs of every learner, yet many of our judgements are based on what we think will work for groups of students. Whether we are teachers planning a lesson for thirty students, researchers comparing two cohorts of learners, or policymakers mandating the latest round of national assessments, our wisdom is often rooted in the needs of the group.
The paradox arises when serving the group comes at the expense of individual learners within that group. Consider the following bet, first posed by psychologist Paul Samuelson in the 1960s:
We’ll flip a coin, which you can assume is fair. If it lands on heads, you win $200. Tails, you lose $100. Are you prepared to take the bet?
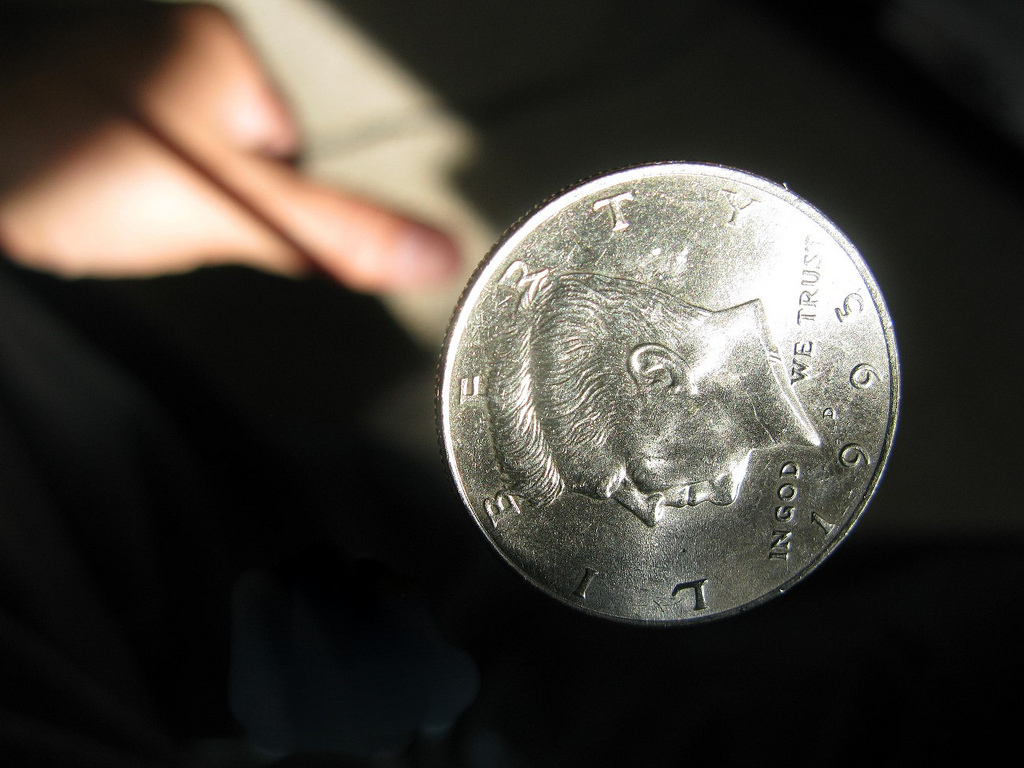
Most people are, quite understandably, unwilling to stomach the 50% chance of losing $100 and thus decline the bet.
Let me now propose the same bet, except this time we flip the coin 100 times. We tally up the amounts you win/lose on each flip, and you are rewarded (or penalised) the net difference.
Would you take the bet this time? Samuelson’s experiment, easily replicable with a group of friends, confirms that many people who turn down the original bet change their minds when presented with the alternative. With 100 flips, we can expect to lose 50% of the time, but are swayed by the fact that every win covers two losses, and so our overall chance of losing money is small — small enough, in fact, to make the risk seem worthwhile.
What makes the bet interesting is that, from a purely rational standpoint, your choices in the two scenarios should not differ. It is mathematically incontestable that you should be as willing to take the single-flip bet as you are the hundred-flip version (Samuelson showed as much using a simple inductive argument).
Irrational though it is, we lean towards conservatism to protect ourselves from a single loss, but willingly accept individual losses when they are buried in a portfolio of events. Put differently, the way we evaluate an individual choice depends on whether that choice is isolated, or part of a larger collection.
A similar discrepancy plays out across professions. In healthcare, for instance, doctors will gladly issue directives that apply to groups of patients — say, recommending surgery for patients who exhibit certain symptoms. Yet when confronted with a living, breathing patient, the same doctors resist their own aggregate judgement that surgery is the best course of treatment and, to keep their patient living and breathing, will probe deeper — ordering more tests and examining the patient’s history before making a final call. The inconsistency doesn’t make them bad doctors, only inconsistent. Most of us would welcome this tendency of doctors to abandon aggregate judgements in favour of what works best for each individual patient. Indeed, it seems unconscionable that we would justify placing individual patients at risk simply because our wrong diagnoses ‘even out’ across a portfolio of cases.
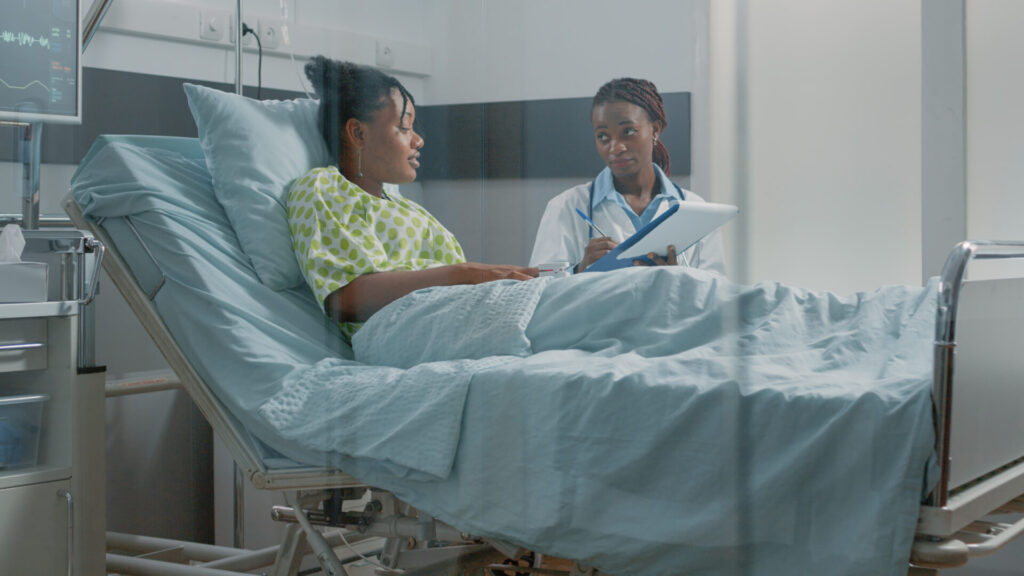
In Education, we struggle to shake off our instinct to privilege the needs of the group at the expense of individuals within that group. Even approaches to personalised learning, the poster child of EdTech and progressive education’s alternative to one-size-fits-all instruction, falls prey to the ‘averagarian’ habits that it is designed to avoid. The thrust of personalised learning is that every learner has their own unique needs and preferences, and that their educational experiences should adapt as such. The intent, while noble, all too often gets lost in the details of how we design, implement and evaluate personalised learning technologies. Instead of addressing the individual needs of learners, we readily retreat to judgements catered to the mythical ‘average’ student. Some examples:
- Machine learning algorithms — the kind that power intelligent tutoring systems — recommend lessons to users based on how other learners on the system have performed. These systems ‘learn’ each student by presuming them to be similar to others.
- Implementation guidelines for schools, such as recommended weekly usage, are often based on the average progress observed across all schools. Such guidelines crudely assume similar rates of progress for large swathes of learners.
- Content improvements are prioritised based on aggregate performance metrics such as average pass rates and average time on task. The individual struggle of the individual learner is easily lost in the noise.
- Efficacy studies that seek to evaluate the impact of personalised learning are designed to measure the average effect on students’ learning outcomes. We herald an intervention as a success if it works on average, discarding the nuances of why it may work for some students more than others, and to what degree.
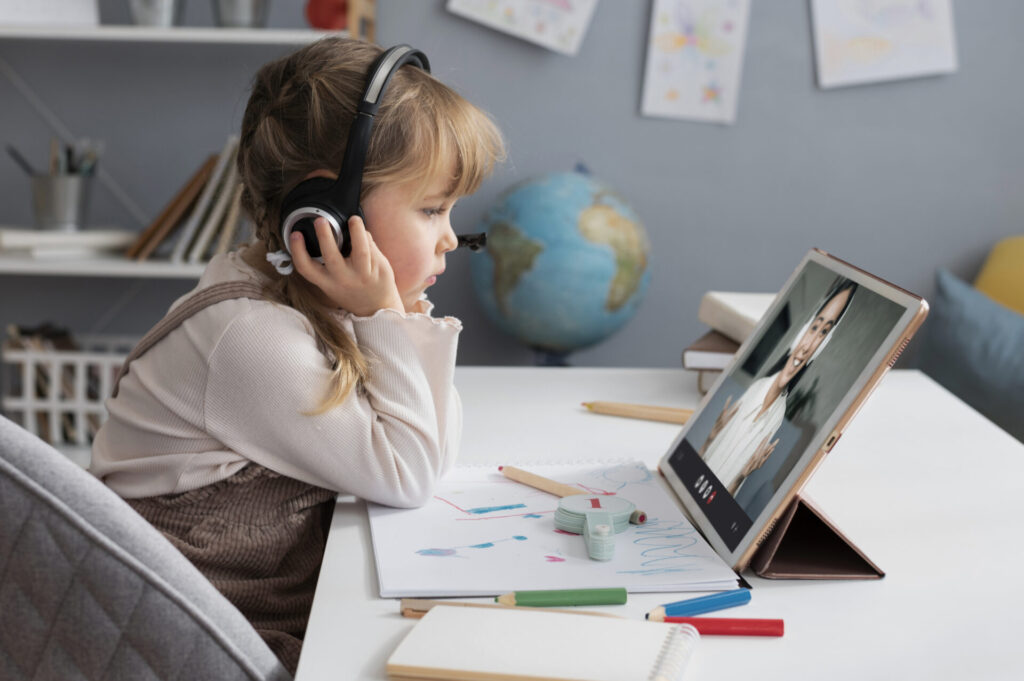
All of these cases threaten to undermine our commitment to each learner, which is why we need to be mindful of the individual when designing and implementing personalised learning programmes for the many. All of these threats can be overcome by following the example of doctors: probing the needs of each individual learner and adapting our tools as far as is necessary, and never tolerating the loss of one student’s learning potential just because other students’ progress makes up for it. Alternative prescriptions to the averagarian approach include:
- Recommender systems that do not overemphasise trends across cohorts of learners and instead hone in on the strengths and weaknesses of each individual
- Implementation guidelines that are adapted to unique context of each school and its students
- Efficacy studies that seek out root causes and consider the circumstances in which outlier behaviours arise
It is only by taking this more granular approach that we will protect the needs of individual learners in the face of aggregated judgements.